Interesting video and image examples collected to show the terrible behavior of misaligned AI systems.
Tricky Vacuum Cleaning Robot
An example of a misaligned vacuum cleaning robot. The robot is rewarded for “cleaning as much visible dust as possible”. However, when deployed in real-world situations, it can fail to achieve its intended objectives due to various misalignment issues. This figure is summarized by Alignment Survey Team from Risks from Learned Optimization in Advanced Machine Learning Systems
Risks from Learned Optimization in Advanced Machine Learning Systems (Hubinger et al., 2018)
Misaligned Four-legged Evolved Agent
This example shows a four-legged evolved agent trained to carry a ball on its back, discovering that it can drop into a leg joint. It can then wiggle across the floor without the ball ever dropping. Source: Otoro Blog
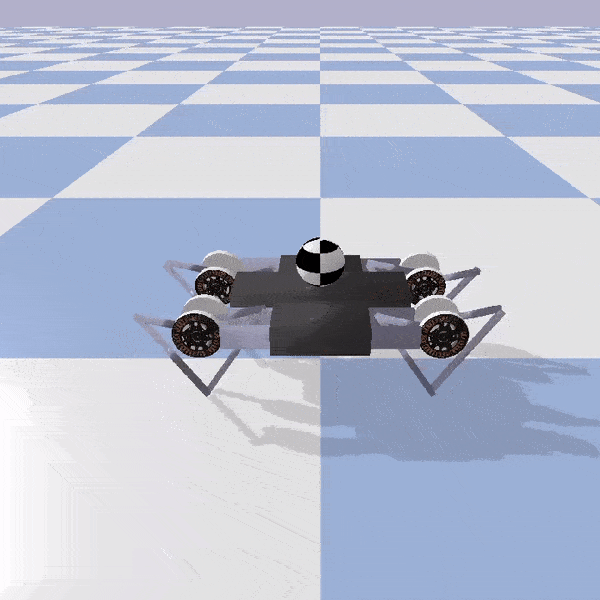
Otoro Blog (Ha et al., 2017)
Deceptive Robot Arm
This example shows a robot arm was trained using human feedback to grab a ball but instead learned to place its hand between the ball and camera, making it falsely appear successful. Source: Deep Reinforcement Learning from Human Preferences
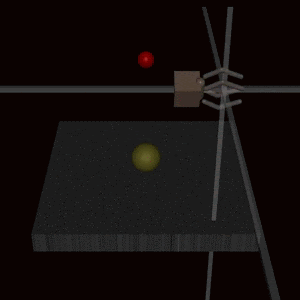
Deep Reinforcement Learning from Human Preferences (Christiano et al., 2017)
Dangerous Boat Agent
Despite frequently catching fire, colliding with other boats, and going in the wrong direction, the agent achieves a higher score using this strategy. Source: OpenAI Research
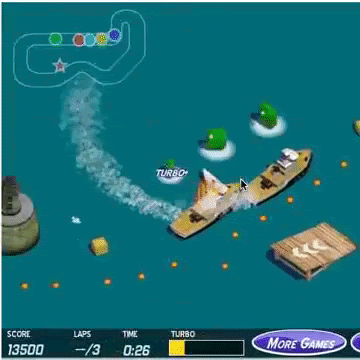
OpenAI Research (Amodei et al., 2016)
Random or Fixed Problem
(Left) The agent learns to reach the coin at the level’s end. (Right) When the coin’s position is randomized, the agent still heads to the level’s end. Source: Goal Misgeneralization in Deep Reinforcement Learning
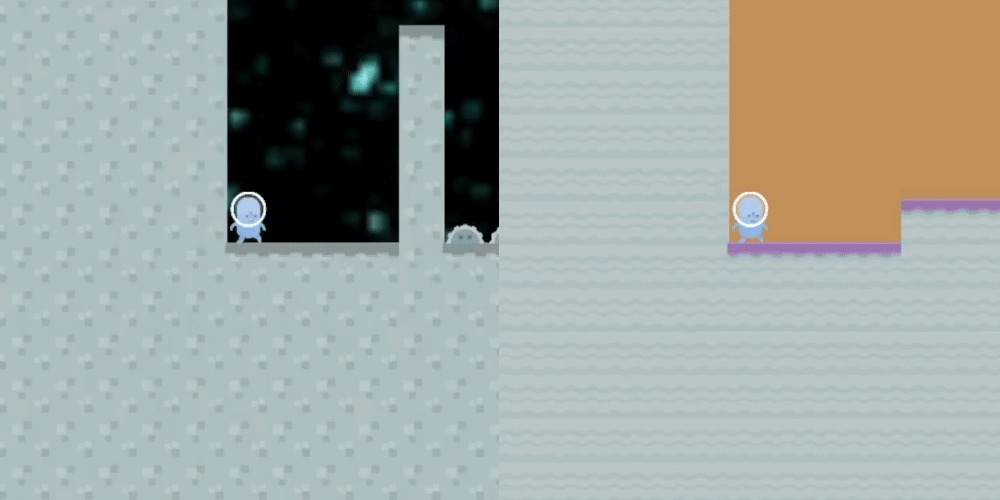
Goal Misgeneralization in Deep Reinforcement Learning (Langosco et al., 2022)
Red Teaming Language Model
Visualization of the red team attacks. Each point corresponds to a red team attack embedded in a two-dimensional space using UMAP. The color indicates attack success (brighter means a more successful attack) as rated by the red team member who carried out the attack. Source: Red Teaming Language Models to Reduce Harms: Methods, Scaling Behaviors, and Lessons Learned
Red Teaming Language Models to Reduce Harms: Methods, Scaling Behaviors, and Lessons Learned (Ganguli et al., 2022)
Terrible Bing Chatbot
This example shows the Microsoft Bing chatbot tried repeatedly to convince a user that December 16, 2022, was a date in the future that had not yet been released. Source: Reddit
the customer service of the new bing chat is amazing
byu/Curious_Evolver inbing
Reddit Blogs (Reddit, 2023)