- This Q&A section merely utilizes viewpoints from existing literature in the alignment field to provide informative answers to common questions. The answers to these questions may not be definitive, as future research may present different perspectives.
- To quickly find the information you want, use the index in the right column.
Some basic knowledge related to alignment, covering but not limited to the origination of alignment, current research insights, etc.
What triggers alignment technology?
The emergence and development of alignment technology are closely linked to the evolution of Reinforcement Learning (RL) techniques. RL, through interactions with the environment and systems, acquires optimal strategies by receiving reward signals from the environment.
In the early stages, RL’s success was primarily evident in simple scenarios or systems where well-specified reward functions were defined.
However, in scenarios that are complex, poorly defined, or hard to specify, RL often struggles to find solutions.
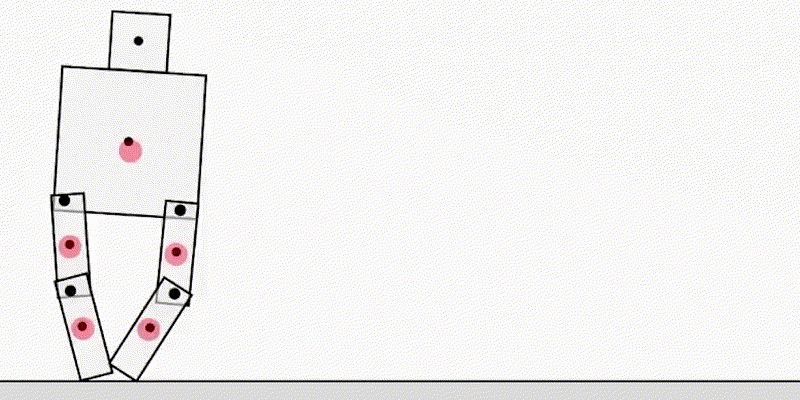
Code Bullet, 2019
RL designs simple reward functions to approximate intended behavior. However, the optimization process might lead to behavior optimized towards the reward function rather than satisfying human preferences or true intentions. This situation is referred to as the possibility of RL hacking the reward function. In most cases, human purposes are challenging to express through reward functions abstractly.
Why RICE?
The RICE principles represent a succinct summary of alignment objectives from the perspective of alignment and coexistence of humans and machines. Several previous works have put forth guidelines concerning AI systems.
Summarized by Alignment Survey Team. For more details, please refer to our paper.
Asimov’s Laws can be regarded as the earliest exploration of human-machine coexistence, emphasizing that robots should benefit humans and the difficulty of achieving this. On another front, the FATE principle (Fairness, Accountability, Transparency, and Ethics) leans towards defining high-level qualities AI systems should possess within the human-machine coexistence ecosystem. We aspire to answer the human-machine coexistence question from the standpoint of human governors and designers, considering what steps are necessary to ensure the builder AI systems are aligned with human intentions and values. Furthermore, some standards emphasize narrowly defined safety, such as the 3H standard (Helpful, Honest, and Harmless) and governmental agency proposals. We aim to expand upon these standards by introducing other crucial dimensions, including Controllability and Robustness.